VE1076 M.2 A+E Key Card
Overview
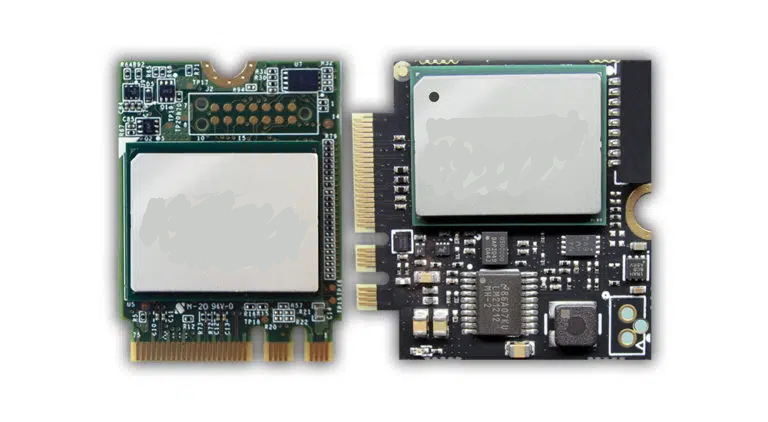
Features
- V1076 Volga AMP™ with support for up to 80M weights on-chip
- No external DRAM required
- SMBus for EEPROM and PMIC access
- Pre-qualified networks including object detectors, classifiers, pose estimators, with more being added
- OS Support: Ubuntu, NVIDIA L4T, and Windows (future release)
- Model parameters stored and matrix operations executed on-chip by AMP tiles
- 2-lane PCIe 2.1 for up to 1GB/s bandwidth
- Support for standard frameworks, including PyTorch, TensorFlow 2.0, and Caffe
- Small 22mm x 30mm form factor
Workflow
DNN models developed in standard frameworks such as Pytorch, Caffe, and TensorFlow are implemented and deployed on the Volga Analog Matrix Processor (Volga AMPTM) using Volga’s AI software workflow. Models are optimized, quantized from FP32 to INT8, and then retrained for the Volga Analog Compute Engine (Volga ACETM) prior to being processed through Volga’s powerful graph compiler. Resultant binaries and model weights are then programmed into the Volga AMP for inference. Pre-qualified models are also available for developers to quickly evaluate the Volga AMP solution.
DNN Model Library
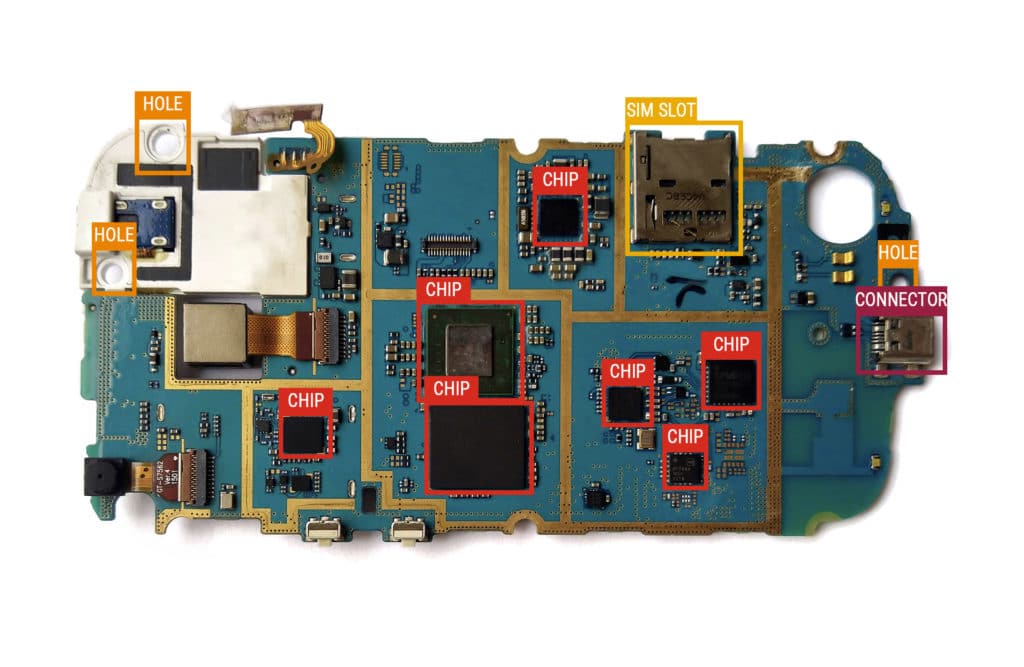
Object Detection and Classification YOLOv3, YOLOv5, ResNet-50, ResNet-18
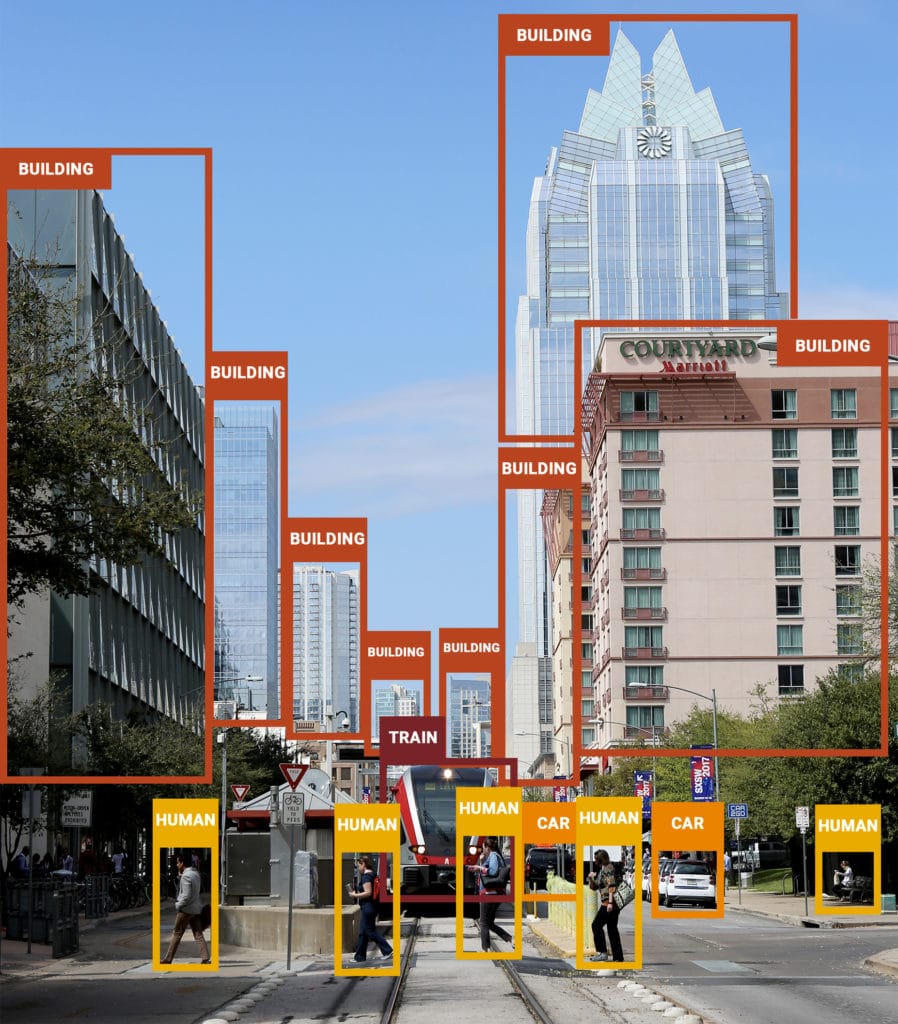
Object Detection and Classification YOLOv3, YOLOv5, ResNet-50, ResNet-18
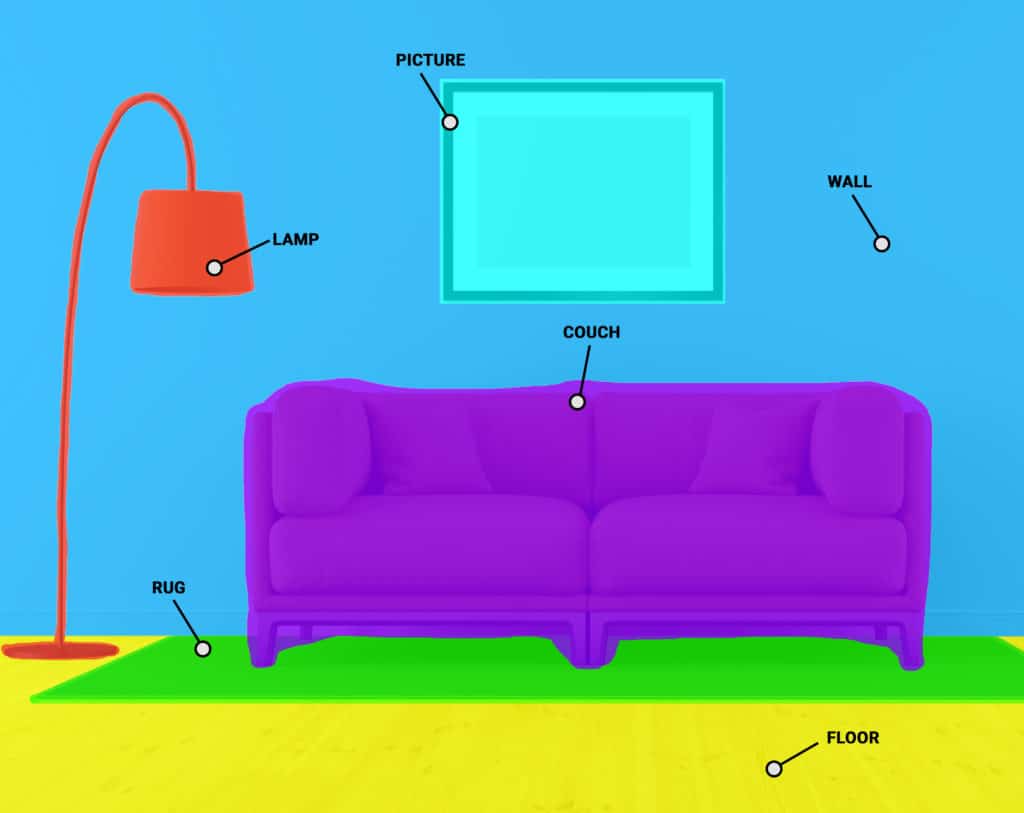
Scene Segmentation SegNet
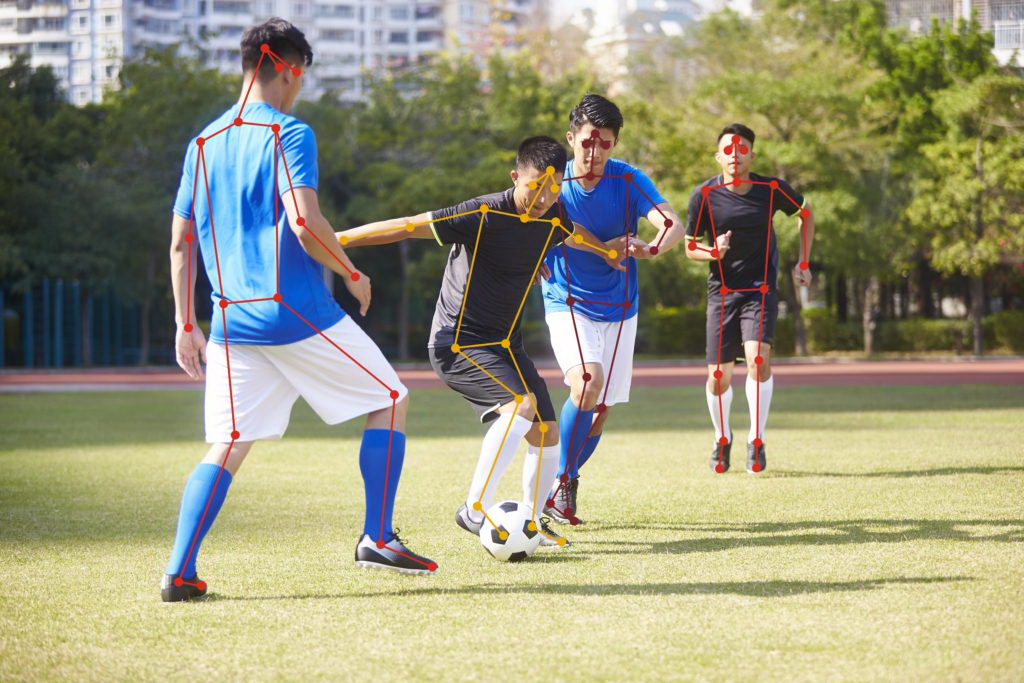
Human Pose Estimator OpenPose Body25