VP10304 Quad-AMP PCIe Card
Overview
The Volga VP10304 Quad-AMP PCIe card enables high performance, power efficient AI inference for edge devices and servers. The half-height, half-length (HHHL) PCIe card simplifies the integration effort into platforms where space is a constraint. The VP10304 features four M1076 Volga Analog Matrix Processors (Volga AMP™), delivering up to 100 TOPs of AI performance and supporting up to 320 million weights for complex AI workloads at less than 25W of power. Large DNN models can be deployed on the VP10304 PCIe card using the combined AI compute of the four M1076 AMPs. The VP10304 can also run a variety of smaller DNN models for video analytics applications processing images from multiple cameras.
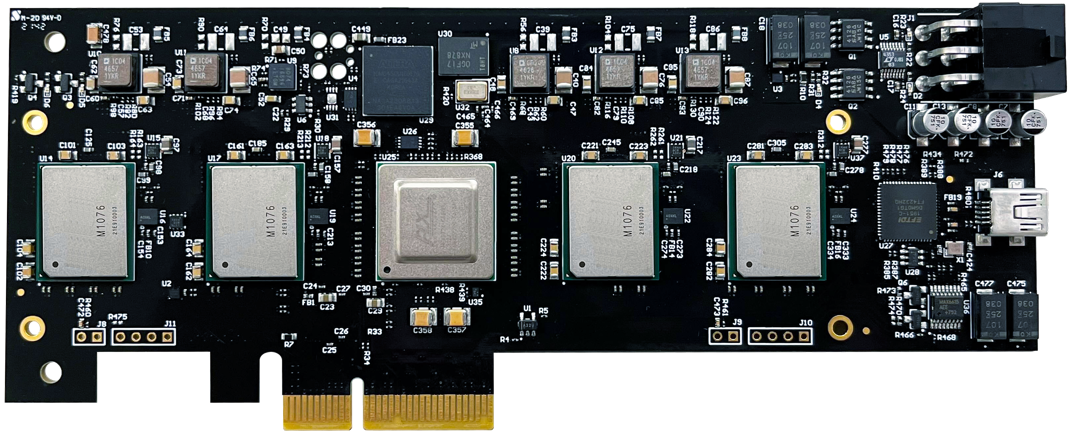
Features
- Four M1076 Volga AMPs
- AI compute performance of up to 100 TOPs
- No external DRAM required
- Support for industry standard AI frameworks
- Pre-qualified networks including object detection, classification, pose estimation, depth estimation, and image segmentation networks
- Support for up to 320 million weights on-chip
- On-chip storage of model parameters
- 4-lane PCIe 3.0 for up to 3.9GB/s bandwidth
- OS Support: Ubuntu, NVIDIA L4T, and Windows (future release)
Workflow
DNN models developed in standard frameworks such as Pytorch, Caffe, and TensorFlow are implemented and deployed on the Volga Analog Matrix Processor (Volga AMPTM) using Volga’s AI software workflow. Models are optimized, quantized from FP32 to INT8, and then retrained for the Volga Analog Compute Engine (Volga ACETM) prior to being processed through Volga’s powerful graph compiler. Resultant binaries and model weights are then programmed into the Volga AMP for inference. Pre-qualified models are also available for developers to quickly evaluate the Volga AMP solution.
DNN Model Library
Volga provides a library of pre-qualified DNN models for the most popular AI use cases. The DNN models have been optimized to take advantage of the high-performance and low-power capabilities of the Volga Analog Matrix Processor (Volga AMPTM). Developers can focus on model performance and end-application integration instead of the time-consuming model development and training process. Available pre-qualified DNN models include: